In today’s competitive manufacturing landscape, integrating Design for Manufacturability (DFM) principles early in the product development cycle is crucial to minimize costs, reduce iteration loops, and ensure high-quality output. However, traditional DFM processes often struggle with complex geometries, siloed collaboration, and costly late-stage design changes. Artificial intelligence (AI) driven tools are now emerging to address these pain points by providing generative design capabilities, digital-twin simulations, and predictive analytics that embed manufacturability checks directly into CAD environments. This article explores the main challenges engineers face in DFM, surveys how AI is transforming DFM workflows, and profiles five leading AI-enabled tools, detailing their features, benefits, and limitations.
Key Challenges in DFM
Complex Geometries and Feature Interactions
Modern products increasingly demand intricate shapes, such as lattice structures, tight fillets, and undercuts, that push the limits of conventional machining and molding methods. Each added feature can require specialized tooling or additional setup operations, driving up both cycle time and per-part cost. In injection molding, sharp corners and sudden thickness changes can introduce flow imbalances, leading to sink marks, voids, and warpage, issues often detected only after expensive tool fabrication.
Cost, Cycle Time, and Iteration Bottlenecks
CNC machining costs scale with machine hours, number of tool changes, and the flips (operations) required to access all surfaces. For small batches, setup time can dwarf actual cutting time—sometimes a single flip setup may take an hour, compared to minutes of machining. Likewise, injection-molded prototypes may require multiple mold-flow simulations or trial runs, each taking days and inflating budgets. Late-stage DFM corrections after tooling design can delay time-to-market by weeks or months.
Data Quality and Cross-Functional Collaboration
Traditional DFM reviews often occur after detailed CAD models are completed, creating siloed handoffs between design and manufacturing teams and delaying feedback. Poor version control or incomplete manufacturing specifications can lead to discrepancies—such as mismatched tolerances or material requirements—forcing rework and undermining first-pass yield.
Along with above challenges the following challenges creates more complication in a long-run.
Design-Process Misalignment
Features like holes or slots placed too close to bends cause distortion during fabrication. Non-standard bends or sharp corners increase tooling costs and risk material stress. And Mismatched materials (e.g., using stainless steel for lightweight applications) lead to performance failures or inflated costs.
Tolerance and Quality Control Gaps
Tight tolerances (±0.1mm) where unnecessary escalate costs by 3–5x. Manual checks often miss critical issues like solder mask misalignment or thermal relief errors.
Collaboration and Knowledge Silos
Designers and manufacturers often lack real-time feedback loops, causing delays (e.g., PCB designs failing due to unmarked critical surfaces). Reliance on manual PDF specifications or Excel BOMs introduces errors and delays.
Cost and Time Overruns
Finally, Poor material utilization increases scrap rates by 20–30%, and unaccounted surface finishes (e.g., powder coating thickness) force redesigns.
How AI Is Revolutionizing DFM
AI technologies are being embedded throughout the DFM process to provide rapid, automated manufacturability insights and design optimization:
- Generative Design: By specifying performance objectives and manufacturing constraints, AI-driven generative algorithms explore thousands of design permutations, delivering lightweight, functionally optimized geometries that respect machining or molding limitations.
- Digital Twins & Simulation: Virtual replicas of production processes enable real-time DFM evaluations within hours rather than days. Simulation-integrated CAD platforms can highlight manufacturability issues—such as undercuts for molding or impossible toolpaths for CNC—directly in the design environment.
- Machine Learning Predictive Analytics: Historical manufacturing data trains models to predict costs, lead times, and defect probabilities for a given part geometry. Instant quoting engines leverage these neural nets to provide designers with cost-and-time estimates alongside DFM feedback, closing the loop between design choices and real-world production metrics.
- Automated Rule Checking: AI scans designs against 1,200+ DFM rules (e.g., solder pad spacing, drill alignment) to flag issues pre-production
- Cost Prediction: AI calculates real-time manufacturing costs based on material usage, tooling, and labor, preventing budget overruns
- Knowledge Standardization: Platforms like Footprintku convert tribal DFM expertise into structured, reusable rules
Together, these AI capabilities reduce manual checks, accelerate iteration loops, and embed manufacturability intelligence directly into design workflows.
Five Leading AI DFM Tools
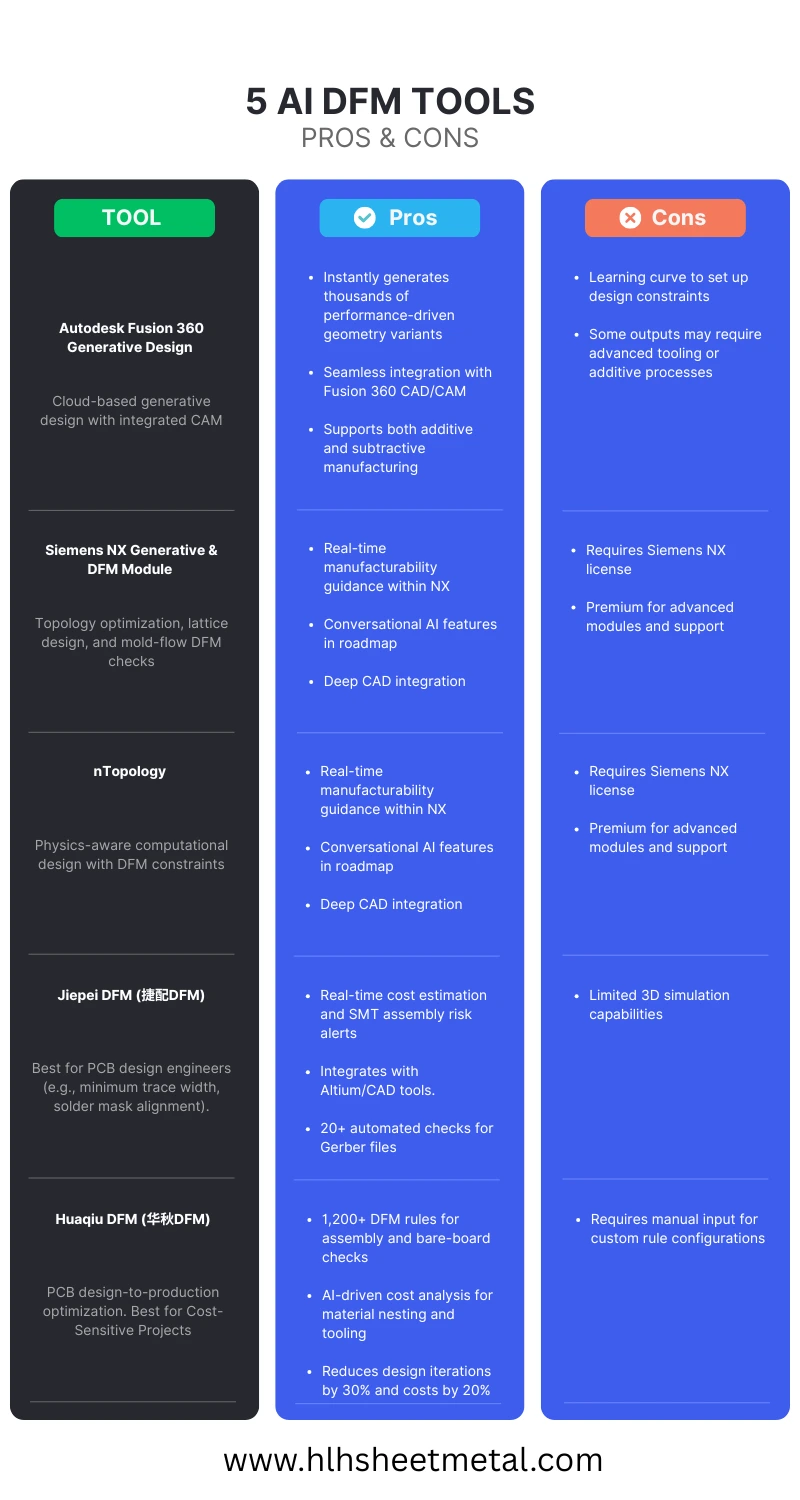
1 Autodesk Fusion 360 Generative Design
Autodesk Fusion 360’s generative design module leverages cloud computing to iterate through design solutions constrained by user-defined loads, materials, and manufacturing methods. Designers receive a spectrum of optimized geometries—each balancing weight, strength, and manufacturability. Because it integrates seamlessly with Fusion 360’s CAM workspace, toolpaths for CNC milling or additive setup for 3D printing can be generated directly from selected designs, collapsing traditional DFM review cycles.
Pros
- Unlimited cloud solves enable exploration of many “what-if” scenarios.
- Generative outputs consider both additive and subtractive processes.
- Integrated simulation tools (FEA, injection-mold flow) provide early validation.
Cons
- Requires upfront investment in CAD training and cloud credits.
- Some geometries may necessitate specialized machining or support structures beyond standard CNC workflows.
2 Siemens NX Generative & DFM Module
Siemens NX embeds AI-driven topology optimization and DFM guidance directly into its CAD environment. Users can run manufacturability checks on molded parts—highlighting draft-angle violations, wall-thickness anomalies, and potential undercut issues—while iterating geometry in real time. Future updates promise conversational AI interfaces to further streamline design reviews.
Pros
- Real-time feedback on manufacturability within NX.
- High-fidelity mold-flow and lattice structure optimization.
- Strong enterprise support and comprehensive CAD/CAM integration.
Cons
- High licensing costs and module-based pricing.
- Steep learning curve for topology and lattice optimization tools.
3 nTopology
nTopology’s computational design platform excels at generating complex internal lattices and topology-optimized geometries. Its “blocks” library includes DFM constraints—such as minimum feature sizes, overhang angles, and surface finish considerations—ensuring that designs adhere to machining or molding capabilities before export. A built-in API allows companies to codify their own manufacturing rules into custom workflows.
Pros
- Powerful for advanced lightweighting and conformal lattice work.
- Rapid iteration with instant manufacturability feedback.
- Extensible via scripting and custom blocks.
Cons
- Pricing may be prohibitive for smaller organizations.
- Initial setup of custom libraries and rules can be time-intensive.
4 Jiepei DFM (捷配DFM)
Jiepei DFM is a professional and efficient PCB manufacturability design analysis tool, first released on Jiepei Electronics' production platform.

Use Case: PCB design analysis (e.g., minimum trace width, solder mask alignment).
Key Features:
- 20+ automated checks for Gerber files, including hole-to-line spacing and copper balance.
- Real-time cost estimation and SMT assembly risk alerts.
Pros:
- Free for engineers, integrates with Altium/CAD tools.
Cons:
- Limited 3D simulation capabilities
5 Huaqiu DFM (华秋DFM)
Huaqiu DFM is a free PCB design for manufacture analysis tool. A PCB design issue detector and solution provider which is easy to download and install, it is build for one-click analyze and export, It also helps to optimize design and cost.
Use Case: PCB design-to-production optimization.
Key Features:
- 1,200+ DFM rules for assembly and bare-board checks.
- AI-driven cost analysis for material nesting and tooling.
Pros:
- Reduces design iterations by 30% and costs by 20%.
Cons:
- Requires manual input for custom rule configurations.
By embedding AI-driven DFM checks directly into design workflows, these tools help engineers identify manufacturability issues earlier, optimize geometries for cost and performance, and significantly reduce iteration loops. As AI algorithms continue to evolve—incorporating more nuanced manufacturing rules, integrating conversational interfaces, and expanding into new processes, DFM will shift from a gate-checking step to a continuous, intelligent companion throughout product development. Engineers should prioritize tools aligned with their industry’s specific needs—whether it’s PCB design, material innovation, or assembly reliability.
Do you need help in Design For Manufacturing (DFM)?
Talk to our experts. Our qualified engineers are always happy to answer your questions.